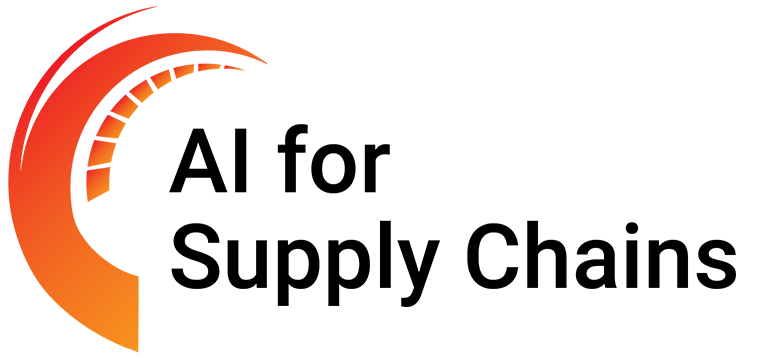
Supply-chain management used to be an arcane topic, discussed by few and invisible to the general public. This has changed since the pandemic: the public is now aware of a topic that has become top-of-mind in many corporate boards. Supply chains have become larger, and e-commerce has proliferated, imposing significant environmental costs to meet new customer expectations. At the same time, many customers and suppliers, especially in rural regions, face increasing difficulties in procuring or delivering specific products. What is needed is a paradigm change, a new vision for supply chains that complements efficiency with resilience, and sustainability goals. Research in supply chains at AI4OPT is centered around end-to-end supply chains, with scalability, resilience, and sustainability as core challenges. AI4OPT has assembled a consortium of partners that cover (almost) all aspects of supply chains. It leverages novel forecasting methods, optimization proxies, decision making under uncertainty, and automation to meet these challenges.
AI4OPT works with partners on all aspects of end-to-end supply chains, from forecasting to optimization and risk management.